Deep Learning Neural Networks in Image Recognition
The Revolution of Deep Learning Neural Networks in Image Recognition
In today’s digital age, we’re surrounded by a staggering amount of visual information. From photographs and videos to medical scans and satellite images, our world is saturated with visuals. Amid this deluge of data, the field of image recognition has seen a revolutionary transformation, thanks to deep learning neural networks. This article delves into the remarkable advancements in deep learning and its profound impact on image recognition.
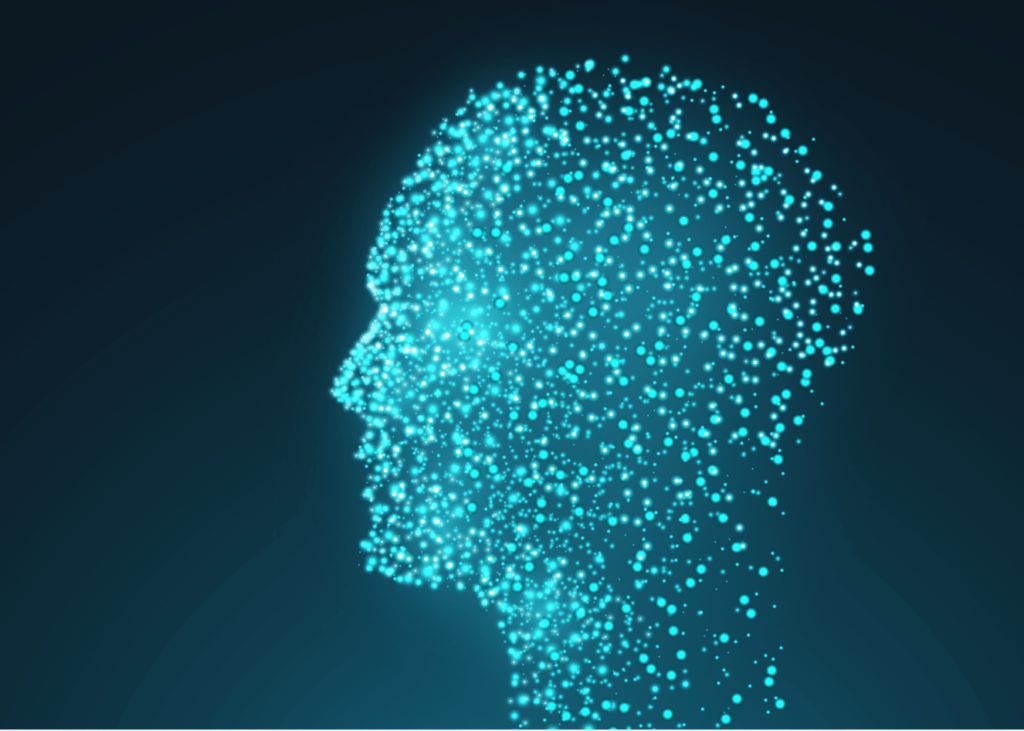
The Evolution of Image Recognition
Image recognition, or computer vision, is the field of artificial intelligence that focuses on enabling machines to interpret and understand visual data. It has applications in various domains, including healthcare, automotive, security, and entertainment. Over the years, image recognition systems have progressed from traditional computer vision techniques to the more recent and powerful deep learning-based approaches.
The Rise of Deep Learning
Deep learning, a subfield of machine learning, has gained prominence due to its ability to automatically discover and learn patterns from data. Unlike traditional machine learning, deep learning neural networks operate with multiple layers of interconnected nodes, mimicking the structure of the human brain. These networks, often referred to as artificial neural networks, have proven exceptionally effective in image recognition.
Convolutional Neural Networks (CNNs)
At the forefront of deep learning for image recognition are Convolutional Neural Networks (CNNs). CNNs are a specialized class of artificial neural networks designed to process and recognize visual data efficiently. They have become the backbone of many image recognition systems.
CNNs work by applying a series of filters or convolutions to the input image, each identifying different features. These features are then aggregated to make high-level representations, allowing the network to identify objects, shapes, and patterns within an image. The remarkable architecture of CNNs has led to their unparalleled success in various image recognition tasks.
Applications in Image Recognition
The applications of deep learning neural networks in image recognition are vast and diverse, revolutionizing industries and enhancing our daily lives. Here are some notable examples:
- Autonomous Vehicles: Self-driving cars rely on deep learning neural networks to detect pedestrians, other vehicles, road signs, and road conditions, ensuring safe and efficient navigation.
- Medical Imaging: Deep learning aids in the early detection of diseases through image recognition of X-rays, MRIs, and CT scans. It can identify abnormalities and assist radiologists in making diagnoses.
- Security and Surveillance: Surveillance cameras use deep learning for facial recognition, object detection, and tracking of suspicious activities, bolstering security efforts.
- Agriculture: Deep learning systems can identify crop diseases, monitor crop growth, and assess soil conditions through image recognition.
- Retail: Image recognition enhances customer experiences by providing real-time product recommendations based on customer behavior and facial recognition for cashierless stores.
- Art and Entertainment: Deep learning is employed to create art, generate special effects in movies, and even restore and colorize old black-and-white photos.
Challenges and Breakthroughs
While deep learning neural networks have yielded remarkable results, they are not without challenges. These include the need for vast labeled datasets, computational resources, and addressing biases in the training data. Researchers and engineers have made significant breakthroughs in addressing these issues:
- Transfer Learning: By leveraging pre-trained models, transfer learning allows developers to adapt existing neural networks to new tasks with smaller datasets, reducing the need for massive data collection.
- Data Augmentation: Data augmentation techniques generate more training data by applying transformations to the existing data, increasing the model’s robustness.
- Explainability: Efforts are being made to make deep learning neural networks more transparent and interpretable, ensuring that the reasoning behind their decisions can be understood.
- Bias Mitigation: Researchers are actively working to mitigate biases in image recognition systems, particularly in applications like facial recognition, to prevent unfair and harmful outcomes.
The Future of Deep Learning in Image Recognition
The trajectory of deep learning in image recognition shows no signs of slowing down. As technology advances and data becomes more accessible, we can expect to see even more impressive developments. Here are some areas of interest for the future:
- Real-Time Recognition: The drive for real-time image recognition, especially in augmented and virtual reality applications, is on the rise. Deep learning will continue to play a pivotal role in making this a reality.
- Healthcare: Deep learning will further enhance medical image recognition, improving diagnostic accuracy and aiding in the development of precision medicine.
- Privacy and Security: The quest for secure and private image recognition systems, particularly in surveillance and biometric applications, will continue to be a priority.
- Multimodal Recognition: The integration of text, audio, and visual data for more comprehensive understanding and recognition of content will become increasingly important.
- Edge Computing: Deep learning models optimized for edge devices will lead to faster and more efficient image recognition without relying heavily on cloud resources.
- Human-Machine Interaction: Developments in human-computer interaction will enable more natural interactions with machines, such as gesture and emotion recognition.
In conclusion, deep learning neural networks have brought about a paradigm shift in image recognition. With their ability to automatically learn and adapt from data, they have opened doors to a myriad of applications and innovations across various industries. While challenges persist, ongoing research and development promise a future where image recognition is not just commonplace but also efficient, accurate, and ethical.
As the technology continues to evolve, the potential of deep learning in image recognition remains limitless. Its impact on our daily lives and the industries we rely on is only expected to grow, ushering in an era of visual intelligence that was once the stuff of science fiction but is now very much a part of our reality.